A big flirt with machine learning
A big flirt with machine learning is one way to capture the essence of the three days of lectures and workshops in statistical learning and data science hosted by Tinbergen Institute and the econometrics department of Erasmus University Rotterdam.
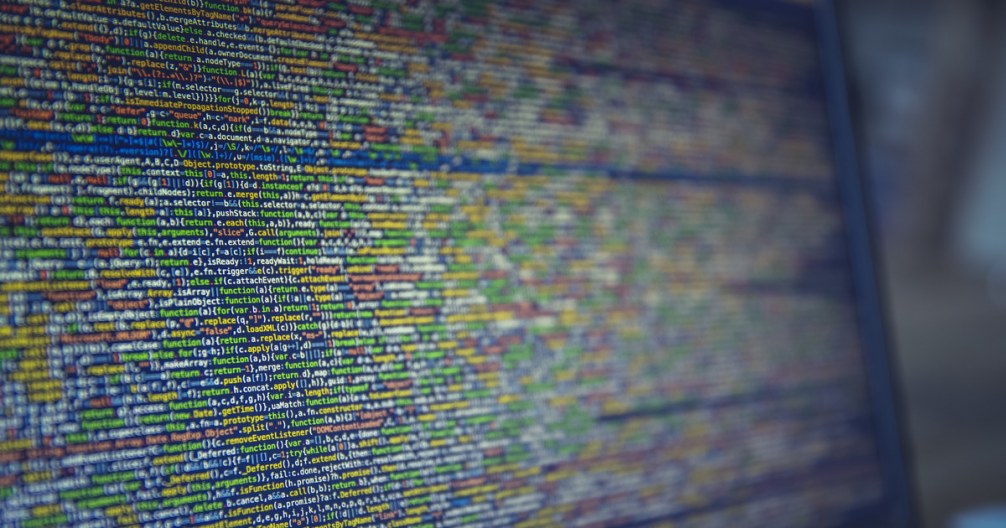
In the first two days, Trevor Hastie, Professor of Statistics at Stanford University, gave the TI Econometric Lectures, in which he provided an overview of models used for Big Data. Most econometricians and economists in the room were already familiar with methods such as Ridge Regression and Lasso, but methods such as bagging and boosting were largely unexplored. Random Forests, neural networks, and deep learning are even more exotic methods.
The fact that machine learning itself lacks a certain familiarity with economics became clear from the list of applications: predicting heart attacks, identifying handwritten numbers and classifying pixels in images. These problems, however, share some properties with recent challenges in economics. Just as in machine learning, in macroeconomics we also work with ‘wide’ Big Data: The number of indicators that can explain, for example, economic growth, increases much faster than the number of observed time periods. In microeconomics, we often observe the behaviour of a vast number of individuals— what Hastie would call ‘tall’ Big Data.
The flirtation was a great success. The number of participants shows that economists are increasingly open to learning from such fields as statistics, computer science and machine learning. But we should not forget that economics also has a lot to sell! Where these fields merely seem to focus on prediction, and do not mind that these predictions are produced by black boxes, econometricians have a great deal of knowledge that may lead to greater understanding about which variables affect the outcome and how. The third day I had the opportunity to present the research I have done with Tom Boot on Big Data from an economic perspective. In the middle of a random forest of workshops, we tried to show that we can use machine learning techniques to improve economic forecasting, and econometric techniques to open the black box of machine learning techniques.