Exploring the world of financial forecasting models
The Tinbergen Institute Econometrics Lectures: Interview with Allan Timmermann.
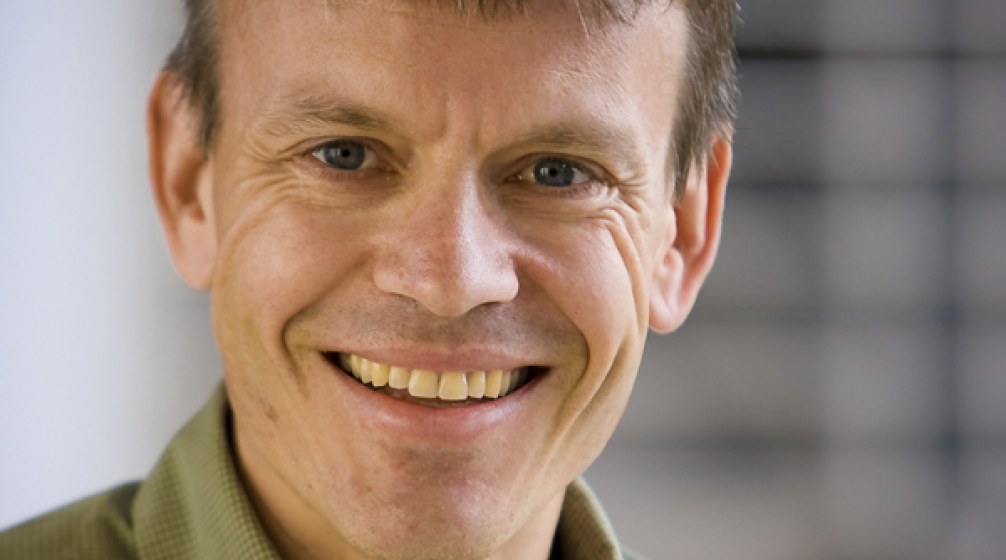
We look forward to your teaching of the Tinbergen Institute Econometrics Lectures this June. What is the main message you would like to send through these lectures? What should students expect?
Well, I certainly hope to get across my enthusiasm for economic and financial forecasting, and especially for the many unique aspects of forecasting economic time series that you do not see in forecasting the weather or forecasting in the natural sciences.
One unique element of financial forecasting is the interaction of economic agents with their forecasts: for example, if agents predict abnormally high future returns on a security, this will lead them to buy the security now, thereby pushing the price up today. Thus, the agents’ forecast may not come true because they acted on it. Some of my more recent work talks about this inherent instability of financial forecasting models, something akin to “now you see it, now you don’t”. For this (and other) reasons, financial forecasting models are likely to be subject to “breakdown” risk.
How do you design forecasting models to be adaptive without being overly sensitive to “noise”? How do you communicate the uncertainty around a forecast? There have been many exciting developments on evaluating and monitoring forecasting performance, which I also plan to talk about.
Forecasting Financial Time Series
With the growing popularity of large datasets, what are the new opportunities for economic forecasting?
This is a golden age for economic forecasting and nowcasting. First, we have seen improvements in forecasting methods. Second, we are getting access to better data sets— some in real-time and at higher frequencies, similar to scanner data from retailers used by marketing researchers. High-frequency indicators are being developed for macroeconomic data. For example, the Philadelphia Fed publishes a daily business cycle indicator for the US economy. Using a dynamic factor model that is updated through a Kalman filtering equation, this allows you on any given day to update your estimate of the current state of the economy and to forecast on the basis of that. I expect more such developments to take place.
Do you think the increased use of machine learning techniques may de-emphasize causality in favor of fit and prediction?
I think this is already happening. A challenge with machine learning methods is that flexible fitting methods sometimes find spurious patterns. One scientist mined Facebook data and found that people who like curly fries had a higher IQ than people who do not. You can use and abuse data for all sorts of purposes— and if you mine a given data set with enough intensity, you are likely to find lots of spurious patterns.
“if used properly and correctly, data-mining methods can be turned to our advantage”
However, if used properly and correctly, data-mining methods can be turned to our advantage. For example, if economic theory tells us that there should be a positive relationship between a predictor variable and some outcome, then we can impose that the machine learning algorithm fits the best model subject to that constraint (or other monotonicity assumptions, etc.). A problem that remains, though, is model selection.
There is work (which I will cover in the lectures) on how to deal with model selection. When you have searched across many possible specifications to find the best model, it is important to adjust your expectations regarding how good the best model or the best set of models should be in order for it/them to remain genuinely significant. For example, a t-statistic of two is not so impressive if you learn that this is the highest t-statistic chosen across 1000 predictor variables.
How can we talk about forecasting returns in an environment where the distribution of prices (and probably also that of the volatility factor) are most likely unknown? How could we overcome these challenges with less strict parametric assumptions?
By and large, for many purposes, if you have a good forecast for the conditional mean and volatility of returns then you capture most of the time variation in the return distribution. However, the conditional mean and conditional variance behave completely differently.
The conditional mean has very little persistence. Most prediction models have very low predictive power. If you can get an out-of-sample R2 at the monthly horizon of half of one percent or one percent, then over time you might turn that into a sizeable economic gain— provided transaction costs are low. Such a small predictive component can easily be overwhelmed by estimation error, however. If you opt for nonparametric techniques you have to be particularly careful in controlling the effect of estimation error and the risk of overfitting. A third issue is that the state variables with predictive content over returns may change over time. As an alternative, you could use techniques from machine learning (such as the LASSO) as a way of selecting predictor variables. But although this method works well in some applications, in time-series applications with short length where the predictive model changes over time, the application of such techniques becomes very tricky.
Returning to statistical learning methods, I will be talking about boosted regression trees. These provide you with flexibility, but can easily overfit and perform disappointingly in out-of-sample forecasting. There are ensemble learning methods for reducing this tendency to overfit by using techniques such as subsampling, shrinkage, or minimizing mean absolute errors instead of mean squared errors.
Turning to the second moment, a large literature has found empirically that if you are interested in forecasting future volatility, current and past volatility (and perhaps also the lagged return and its sign) contain most of the relevant information. So there is weak evidence empirically that you can get much predictive power from variables other than past volatility to come up with significant improvements to your future volatility forecast.
It is difficult to make accurate predictions. Is it perhaps easier to determine the uncertainty around these predictions? Should interval (density) forecasts prevail over point forecasts in applied work?
I am very big on forecasts being part of the decision (-making) process. From that point of view, if I tell you that my forecast for the return in the Dutch stock exchange for the rest of the year is 2%, then the way you will act on this prediction depends on the information about the uncertainty surrounding it. If my 95% confidence interval is between -18 and +22%, or between 0 and 4%, you will act completely differently in the two cases— even if the mean (point forecast) remains the same.
“I am currently working on a paper about monitoring forecast performance: can we come up (in real-time) with test variables that tell us, say, how well a central bank’s forecasting model is doing?”
I think the world is moving towards interval forecasts. I recall that historically, the Bank of England was one of the first institutions to adopt what they call a ‘fan chart’. This exercise implies that at a given, fixed point in time, they report interval forecasts for different quantiles of the distribution, at different forecast horizons (one quarter ahead, two, three quarters ahead, one year, and so forth)— and they use color to indicate uncertainty: red in the middle and more diluted as you move further out. Since then, the IMF has also introduced these fan chart forecasts (for example, in their WEO), as have many central banks around the world. I think that graphs such as the ‘fan chart’ provide an intuitive way to communicate the uncertainty surrounding a forecast.
So, could we use forecast uncertainty to evaluate the quality of a model?
One good thing is that we actually have methods for evaluating how good interval or density forecasts are— by means of probability integral transforms, indicator functions for how often the actual realization lies inside the predicted region (the indicator function follows a well-known process if the model is correctly specified). So I think that we are also becoming better at evaluating whether the degree of uncertainty surrounding a particular forecast is correctly calibrated to the data.
I am currently working on a paper about monitoring forecast performance: can we come up (in real-time) with test variables that tell us, say, how well a central bank’s forecasting model is doing? We have seen in the 90s that some institutions consistently over-predicted inflation and under-predicted economic growth, so an important question is whether we can better monitor and improve these models in real-time.
I guess you are often asked why we couldn’t forecast the 2008 crisis. Why couldn’t we?
Most forecasting models are really adaptive. Take a GARCH model. It does not predict ahead of time that there will be a spike in volatility, but once a spike in volatility hits us on a given day, the conditional forecast of volatility for the next day will be much higher. The GARCH did not predict the initial shock to the volatility, but it will adapt very quickly.
To improve on this, efforts should be made to develop more risk indicators that can quickly warn us of future crises. Stress testing is related to that as well (trying to estimate how prepared banks are under certain counterfactual scenarios). Scenario analysis— or conditional forecasting— is another way of getting an idea of how a crisis could potentially evolve.
Theory
The basic models of asset pricing are elegant (CAPM), but not realistic in their fit of the data. What is the biggest challenge for theoretical models; what features should these incorporate so that they can fit the data?
In practice, you do not know whether return predictability comes from market inefficiency (whether money is left on the table), from a time-varying risk premium component, or from incomplete learning.
Once it comes to estimating and testing the CAPM, there are several difficulties— such as coming up with a good proxy for the market portfolio, accounting for a variety of constraints on investors’ trades, heterogeneity in beliefs, etc. This gives us good reasons to believe that a single-factor model may not be the best possible asset-pricing model. Examples of empirically driven findings that have become standard in the finance profession are the Fama-French factors and the momentum factor.
To the greatest extent possible, empirical work should be guided by economic theory so as to reduce the risk of data mining. Sometimes economic theory is not that conclusive, however. Standard textbooks predict a positive risk-return trade-off (between the conditional variance and conditional mean). It turns out, however, that it is very easy to extend standard asset-pricing models to obtain a non-monotonic relationship between the conditional mean and variance of returns. Instead, the relation can be upward-sloping, downward-sloping, U-shaped, inverse U-shaped, etc.
I have some work together with Alberto Rossi from the University of Maryland where we use boosted regression trees to explore the risk-return trade-off. We find that the conditional mean-variance relation is only increasing at low levels of conditional variance. Once you have very high levels of conditional variance, the relationship reverses and becomes negative.
Related to this point, the pricing kernel under risk-neutral pricing (complete markets) may result in unrealistically high prices. Could existing asset-pricing models accommodate more realistic features such as incomplete markets?
Incomplete markets are undoubtedly important: households cannot insure against bad luck in the labor market, and such bad luck can strike at the same time as the stock market gets hit. Larry Schmidt— a former student of mine who is now at the University of Chicago— has some interesting work on this topic.
“I think work on dynamic panels will be a very fruitful area. For example, how do you forecast in a situation with a very large cross-sectional dimension and a small time-series dimension?”
Another point I am interested in is incomplete learning: agents are trying to price assets, but they are doing so in a world that is in flux. They do not know the true state variables, and data do not give them a lot of statistical power to establish good predictive models, etc.
Developments in the field
In your view, what are the main directions of evolution in the field of forecasting? What are the areas where theoretical econometrics has more room to contribute?
I think there is very interesting and exciting work on using not only time-series information but also cross-sectional information to improve forecasts. I think work on dynamic panels will be a very fruitful area. For example, how do you forecast in a situation with a very large cross-sectional dimension and a small time-series dimension?
In the presence of many predictors with weak power, what kind of forecasting techniques work well? Some variables are often clearly relevant, while others are clearly irrelevant— but there can be a gray zone with variables associated with medium-sized t-statistic. These variables have some information, but do not seem to have strong predictive power. Should we use model averaging, principal components or should we apply some other type of dimensionality reduction for these variables?
Academic experience
You have experienced the academic environment in Europe (Cambridge, Aarhus, LSE) and the US (US Fed, IMF, UCSD). What is an important difference between the two that researchers could benefit from knowing about?
People value the academic freedom that is associated with the US system, myself included. You have a lot of time to do research. This is of course also a reason why so many top universities are in the US.
“My advice to graduate students? Don’t be afraid to do things. Even if later something turns out to have been obvious, if it’s not obvious at the time, then ask the questions. And persevere in trying to answer them.”
When people come here as visitors to UCSD it is not just for the weather. It is also because it gives them a chance to dedicate themselves to consistently work on one or more ideas while being able to get feedback from interested colleagues.
What is the most memorable, important thing you learned as a graduate student? What is your advice to graduate students?
As a student I obtained a scholarship to go to Cambridge for three years to do my PhD, and there I was very lucky to get to work with Hashem Pesaran, a world-famous econometrician and outstanding supervisor. So, luck played a role for me. Learning from peers and colleagues was also important to me. I remember meeting on a weekly basis at Trinity College to go through the chapters of Darrell Duffie’s textbook in Steve Satchell’s office.
When I look at students here at UCSD, I see a notable cohort effect. If they start having weekly meetings where they present their own paper, or other people’s papers, start discussing their ideas with each other, they often obtain a cluster of excellence effect. This is one thing I have seen work really, really well.
My advice to graduate students? Don’t be afraid to do things. Even if later something turns out to have been obvious, if it’s not obvious at the time, then ask the questions. And persevere in trying to answer them.