KVS Tinbergen Lecture: Sendhil Mullainathan (University of Chicago, United States)
On Friday November 20 the annual KVS Tinbergenlezing was held by Sendhil Mullainathan (University of Chicago, United States) on the topic of How Machine Learning Can Inform Economic Analysis and Policy: The Use Of Micro-Prediction.
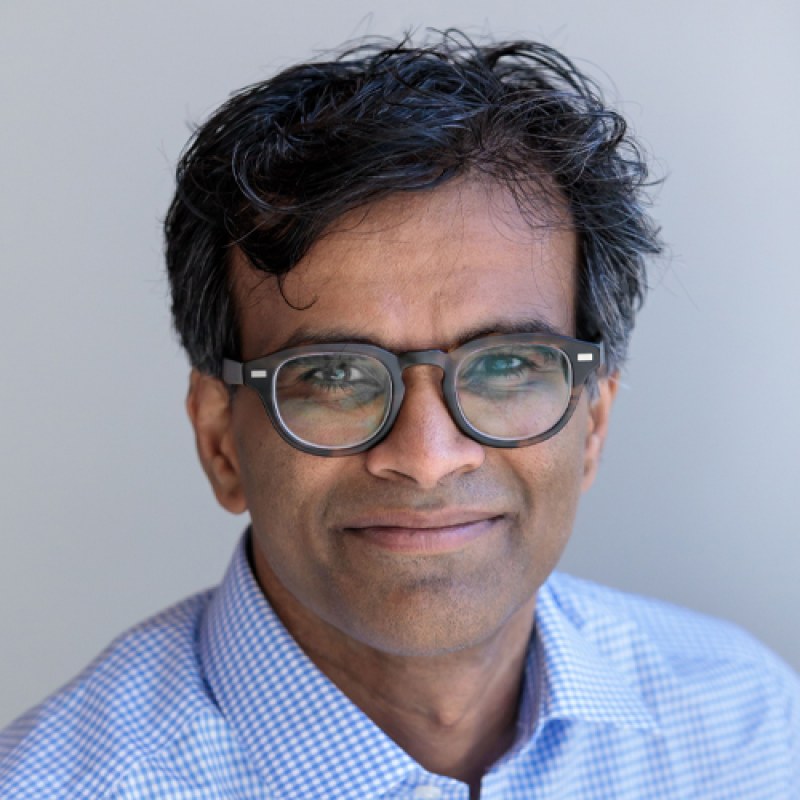
This lecture was part of the Nederlandse Economenweek (www.econweek.nl), which this year took place online November 16-20.
Watch the lecture on youtube.
Short biography
Sendhil Mullainathan is the Roman Family University Professor of Computation and Behavioral Science at Chicago Booth. His current research uses machine learning to understand complex problems in human behavior, social policy, and especially medicine, where computational techniques have the potential to uncover biomedical insights from large-scale health data. In addition to being a co-PI at the joint Berkeley-UChicago Laboratory for Systems Medicine, Sendhil is the cofounder of the computational medicine initiative, Nightingale.
In past work he has combined insights from economics and behavioral science with causal inference tools—lab, field, and natural experiments—to study social problems such as discrimination and poverty. Papers include: the impact of poverty on mental bandwidth; how algorithms can improve on judicial decision-making; whether CEO pay is excessive; using fictitious resumes to measure discrimination; showing that higher cigarette taxes makes smokers happier; and modeling how competition affects media bias.)
is the Roman Family University Professor of Computation and Behavioral Science at Chicago Booth. His current research uses machine learning to understand complex problems in human behavior, social policy, and especially medicine, where computational techniques have the potential to uncover biomedical insights from large-scale health data. In addition to being a co-PI at the joint Berkeley-UChicago Laboratory for Systems Medicine, Sendhil is the cofounder of the computational medicine initiative, Nightingale.
In past work he has combined insights from economics and behavioral science with causal inference tools—lab, field, and natural experiments—to study social problems such as discrimination and poverty. Papers include: the impact of poverty on mental bandwidth; how algorithms can improve on judicial decision-making; whether CEO pay is excessive; using fictitious resumes to measure discrimination; showing that higher cigarette taxes makes smokers happier; and modeling how competition affects media bias.