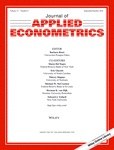
Juodis, A. (2022). A regularization approach to common correlated effects estimation Journal of Applied Econometrics, 37(4):788--810.
-
Affiliated author
-
Publication year2022
-
JournalJournal of Applied Econometrics
Cross-section average-augmented panel regressions introduced by Pesaran (2006) have been a popular empirical tool to estimate panel data models with common factors. However, the corresponding common correlated effects (CCEs) estimator can be sensitive to the number of cross-section averages used and/or the static factor representation for observables. In this paper, we show that most of the corresponding problems documented in the literature can be solved once cross-section averages are appropriately regularized, thus extending the applicability of the CCE setup. As the standard plug-in variance estimators are not able to account for all sources of estimation uncertainty, we suggest the use of cross-section bootstrap to construct confidence intervals. The proposed procedure is illustrated both using real and simulated data.