Markets with Asymmetric Information
An interview with Jonathan Levin and Liran Einav.
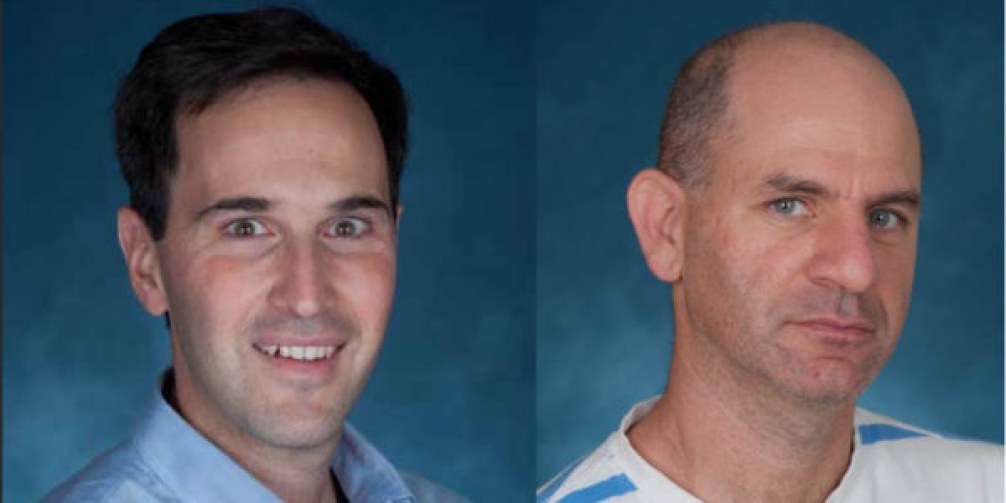
Professors Levin and Einav, according to the Tinbergen Institute course flyer, the series of lectures you gave at TI was on “market failures and inefficiencies due to asymmetric information”. So an obvious first question would be: what got you interested in this topic?
“Actually, now that really terrific data are available and a fair amount of empirical work has been carried out in this context, there are aspects of the theory that appear limited, which means that some empirical results could spur on new extensions and modifications to the theory.”
LE: In my case, I actually got to this area somewhat randomly. In my first year at Stanford, a former classmate from graduate school approached me and asked if I would work with her on a project that
Why focus more on the empirics as opposed to the theory? Is it just that theory has been relatively well developed already, whereas empirical research in the area still faces larger challenges?
JL: The theory of asymmetric information is one of the major contributions of 20th century economics. Market failures due to asymmetric information are commonly used to motivate the regulation of major industries, from banking and finance to insurance to healthcare, and many product and professional service markets as well. It’s also an area where economists spent decades developing the theory and its implications with less concerted effort on empirical evidence and measurement. Nowadays a great deal of data is available to look at markets where we think there might be big imperfections due to asymmetric information— so there’s an opportunity to try to come at all the old questions in a fresh way.
“Information technology has had an enormous effect on almost all of the industries that we think of as having serious informational asymmetries.”
What are the fundamental challenges that are specific to your area of research interest?
Are globalization and the innovations in information and communication technology helping in reducing the (negative) effects of asymmetric information in markets or, to the contrary, is the ICT revolution making these issues more pressing than ever?
JL: Information technology has had an enormous effect on almost all of the industries that we think of as having serious informational asymmetries. To take just one example, the traditional concern in insurance and credit markets is that consumers know much more than the firms offering insurance or loans— but that’s not the case anymore. Nowadays, insurers and lenders often have incredibly data that arguably flip the problem around and make them better forecasters than individuals are.
“The crisis was quite a complicated chain of events… It is probably hard for a single researcher to fully understand all its pieces, and we doubt that any single theory would suffice to explain it.”
It seems all too obvious that we should ask what your opinion is regarding the importance of the topics you have at heart in explaining the crisis that started in 2008. After all, wasn’t it to a large extent an insurance and credit crisis due to asymmetric information (and wrongly designed incentives)?
JL: The most common view among economists is that the crisis was brought on by excessive lending and household leverage. Why did this happen? Most textbook theories of credit markets will actually try to explain why the market will under-provide credit to borrowers, not over-provide it. You can come up with theories where lending is excessive for various informational or incentive reasons (and no doubt there were plenty of incentive problems in credit markets in 2007), but our own view is that it’s hard to understand something like the housing and mortgage boom in the US in the 2000s without moving somewhat outside of the standard optimizing rational expectations models we use in economics.
Turning now to your coverage of the empirical analysis of big data, could you perhaps first explain to the non-specialists: 1) what is meant by the term “big data”; 2) what is special about big data; and 3) what specific challenges do researchers face in this area?
Big data are not specific to economics. Yet, economists have arguably developed and are developing still research tools that could give them an advantage over other professions when it comes to big data analysis. What is your take on this possibility? Are there any red lines we should be wary of crossing? Is collaborative research with scholars from other relevant fields a strategy to defend and advocate?
If we turn to outright violations of the usual codes of integrity, in the last few years, and in the Netherlands as well, there have been a few important cases of such wrongdoings (plagiarism and fraud for example). Regarding the latter, it seems that empirical research is more susceptible to fraud (data massage, non-random data selection, non- or misreporting of results, etc.) than theory is. Do you share this view? What can we do about these issues?
happen very often. At the same time, anyone who has done empirical research in economics understands that it involves dozens or hundreds of judgment calls about how to construct samples, deal with data quality issues, specify regression models, choose what to report and so forth. That’s why, at the end of the day, when you read an empirical paper you inevitably have to assess whether you think the authors are being transparent and making good decisions, and whether the results square with other evidence. You’ve also got to rely to some extent on the authors’ reputations as scholars.