Adapting to the New Reality
An Interview with Christopher Sims.
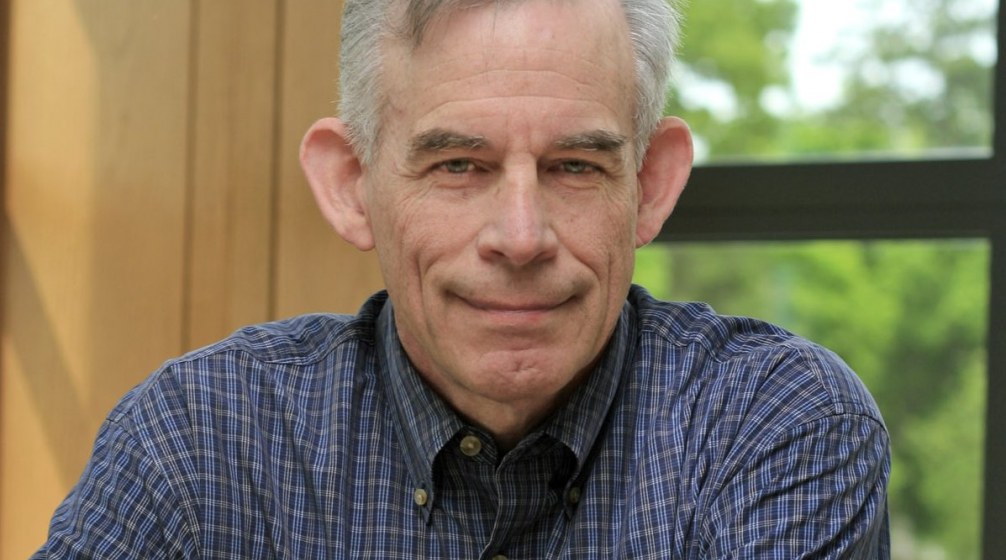
Thank you very much for this interview. As a start, we would like to ask you about the Nobel Prize. What would you say was your core contribution that motivated the Nobel Prize decision? Did you ever think about winning this prize?
What I got the prize for was mostly structural vector autoregressions and their use to understand the effects of monetary policy. Sargent, who shared the prize with me, did some empirical work and a lot of history and theory, and I developed the statistical methods for unravelling the causal effects of monetary policy. Beforehand, people constructed lists on the web of who was likely to get the Nobel Prize. I had never looked at those, but my wife did. Actually, my name had gone up for a while and then it had started to go down. To be honest, I thought it was very likely I would never get the prize, although there was a possibility. The morning of the announcement the phone rang really early— and we didn’t answer it on time; we initially thought that it must have been somebody trying to reach the wrong number. And then I remembered: “This is the morning when the Nobel Prize gets announced, it might be why somebody is calling us at 5:00 a.m.”
How would you describe the process of making research more accessible for the general public? How important is it for academic work to relate to policy and be able to offer advice?
I think it is important for academic research to eventually connect to policy. But there is no simple way of explaining technical economics ideas in ways that the broader public or policymakers or individuals can easily understand. I think it is a matter of understanding the theoretical point well enough so that you can think about it from many points of view— and then find examples or simplifications that make people understand the basics without having to go through the technical details that led you to those insights. It is important for academics who have a knack for it to spend at least some time explaining policy issues to the public— although it can seem like a never-ending task: in policy work and policy-related discussions I often feel that I am repeating myself. But I think that if you want to have an impact on policy discussions you’ll have to repeat yourself—preferably not in exactly the same words or with the same examples—but you have to come back with the same point enough times so that you start seeing other people picking up the insights and repeating them. If you just say once: “This is the truth; everybody should understand that”, it just sinks and nobody listens to it. People need to hear it again and again, and they need to hear it spreading amongst other people, so they don’t just hear one person saying it.
Regarding current developments in monetary policy, what is the potential risk of quantitative easing laying the ground for a new bubble?
I don’t really think there is much danger of a bubble stemming from current policy. What we didn’t do before the crisis was to regulate intelligently. This was not because no one saw that there were problems; it was in good part because some people had strong economic interests in not resolving those problems. In the US there was great enthusiasm for financial deregulation and, as everybody knows, Alan Greenspan had faith that markets would recognize all risks and account for them—and that deregulated markets with a rich array of assets would become a shock absorber and make the likelihood of financial crises much lower. It turned out that that wasn’t true. Not even the research staff at the Fed fully understood how fragile the financial system would be in the face of a major decline in house prices. But now we understand it; we have new institutions that are set up in the US to critically examine the financial structure, and to make sure that large financial institutions don’t end up with balance sheets that could threaten the system under major shocks. So I think it is much less likely that we will have these problems.
Do you think we need a novel, more inclusive way of doing monetary policy— one that also looks at financial markets?
If you want to think of financial regulation as part of monetary policy, it is true that people were not paying enough attention to it before the crisis. The Fed could have paid more attention to it before—and going forward now, the Fed should pay more attention to financial regulation. But some people take the view that monetary policy, in the sense of interest rate policy (the Taylor rule and the like), should now start including arguments that are measures of financial fragility, balance sheet variables, leverage—with the suggestion that raising interest rates when leverage goes up might be a good idea. I don’t think we have models that say this is true, it is basically an instinct that comes out of people having observed that interest rates were low before the crisis and leverage was high—and then we had a crash. So people think that raising interest rates more sharply earlier might have fixed that—but nobody knows for sure, and I don’t know of a causal mechanism that would explain why that would work. There are some models in which raising interest rates in a bubble-like situation actually accelerates the bubble. Certainly, it is likely that if you raise interest rates every time leverage goes up, you will create recessions that didn’t have to exist (because leverage sometimes goes up because there is an investment boom justified by new opportunities).
When it comes to empirical work in economics, it is inevitable that causality will be questioned. Do you believe in causality when you do empirical work in macroeconomics?
The question is what causality means. Of course there is such a thing as causality. It is one way of talking about policy actions: unless we’ve misled ourselves into believing that policy actions have no effect, there are going to be effects of policy actions. The problem is to figure out what the effects of the policy are by looking at historical data. In the experimental sciences (some of psychology, some of physics, most of chemistry), the theories can be tested by generating data from experiments. In economics, we do some experiments— but they are not really relevant for the big macroeconomic policy issues. The experiments people can run are mostly about very micro-policy interventions— and even there, it is really difficult to translate the economic experiments to results that are applicable to economywide or country-wide policy. In macroeconomics we cannot do experiments, so we are always looking at data that has been generated by disturbances of many kinds, not all of which are policy. And then there is the question of correlation versus causation: just because we see things moving in the same direction, does that mean that we can interpret that as the effect of A on B, or B on A? How do we separate those things out? And it’s a difficult question. In monetary policy, we’ve gotten some way towards sorting those things out in normal times, when central banks are doing interest rate policy. But for fiscal policy it is much harder: we have people doing simple regressions to try to estimate multipliers, but there are difficult questions about feedback in any of those frameworks. So I certainly believe in causality, in the sense of questioning whether what we observe reflects causal relationships and how you separate causal relationships of different kinds from observed statistical associations. I believe in causality, in that sense.
Seeing that you are an advocate of Bayesian techniques, do you think that in the future these will gain in popularity relative to frequentist econometrics?
I think so. You see it already among statisticians, and you see it already in economics: statisticians who remain focused on abstract, theoretical work find the field kind of running out from under them. Young statisticians almost all collaborate with applied people from various fields (from genetics to astronomy), people who were analyzing huge datasets. They use some methods that just involve computer scientists figuring out how to apply algorithms on a big dataset and finding patterns, and not thinking about inference in the formal sense at all. And I am afraid that econometricians—because they resisted Bayesian methods and tend to be kind of snooty about big data and the new wave of people touting big data— may find themselves becoming irrelevant if they don’t start adapting and using more Bayesian methods and start thinking about all the issues that come up when you have very large, complex models for big datasets. People may want to have a model that extracts some regularities from the data and doesn’t bother to be perfect. But we don’t have much theory about how you do that correctly. You can certainly get led very far astray by taking the computer science approach that ignores the question of whether what they are finding is significant or whether the model is true—but econometricians could make the mistake of not adapting to this new reality.
Given your broad expertise, what do you see as the major future challenges for macroeconomics and econometrics?
I don’t have very unusual views on that. I think everybody understands we need to do a better job at modelling financial- real interactions. There are many people working on that; it is a very difficult modelling task because most of the time financial variables and measures of financial stress have little relation to what goes on in the rest of the economy. And it’s because of that that people could ignore it for so long. Now we know we shouldn’t ignore it—but it is still true that there is not much data to use to estimate these things, because crises happen rarely, and when it is not crisis time these measures of financial stress don’t have a very reliable connection to macro. If you use data up to 2007 for measures of financial stress and put into your SVAR, you may find something—but if you ask how much including those things helps you to forecast, the answer is “not very much”. If you ask, “what does the model say the effects would be of a big shock to the financial sector”, maybe it’s pretty big, but it is not statistically very strong. There is a well-known paper by Bernanke, Gertler and Gilchrist that laid out a model with collateral constraints before the crisis. They didn’t emphasize this in the introduction to their paper, but if you read through the paper they do carry out an exercise where they look at what happens in the case of a financial shock: it’s bad! But they didn’t put a lot of emphasis on it because nobody thought that was a very likely scenario. As for general equilibrium models, the notion that the probability of a big crash matters even when you go through long periods with no crashes is true—but it is very hard to estimate. The rational expectations framework that we’ve been using assumes that everybody has about the same probability model of the economy because they’ve been looking at data for a long period. But 100 years of data doesn’t tell you much about the probability of big crashes: in the US, we’ve had two: the Great Depression and the Great Recession. It is not going to be easy to build a model where everybody has the same opinion about what the probabilities of these things are and about how these probabilities move over time. So, one way to think of it is that Bayesian methods are going to play more of a role if you are actually going to have quantitative models. This is because there is no way to say that the data are going to tell you what everybody should believe about the probability of a crash. You have to say there are different possibilities, so when you estimate you have to allow for the fact that the data are going to leave you uncertain about the probability of the crash, even if you’ve got 50 years of data. And then you have the theoretical problem, which is way deeper. The rational expectations insight is that everybody should have approximately correct expectations because if they hadn’t they would have made mistakes, realized this and revised them. But there is no way people could have realized they made mistakes and revised and had an accurate probability model for the crash of 2008-9. And as we go forward we don’t expect another one of those soon. The rational expectations assumption that everybody shares the same beliefs about this just doesn’t work. And if people have different beliefs about the probabilities, and they are interacting in financial markets, this has big consequences that are difficult to model, with difficult answers to policy questions.