Causal Inference and Machine Learning
Interview with Guido Imbens (The Applied Econometrics Professor and Professor of Economics at Stanford Graduate School of Business, United States).
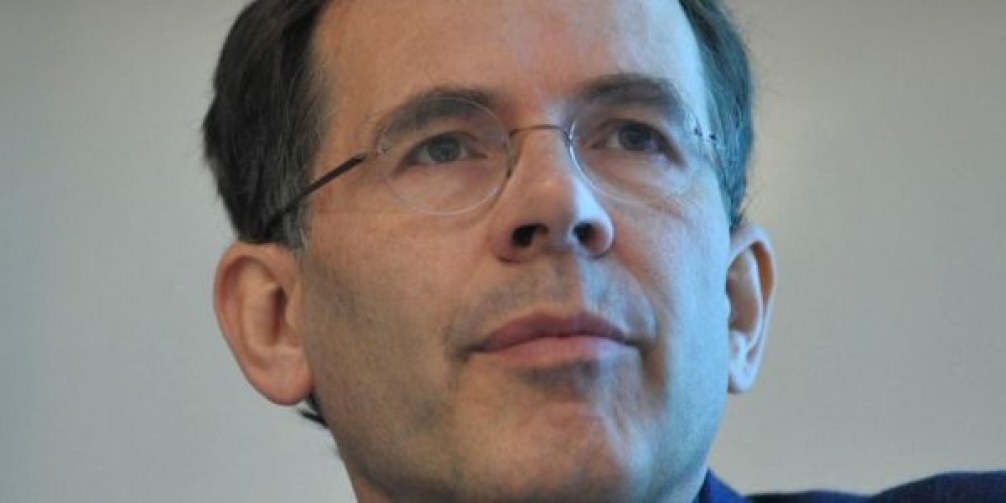
Interview with Guido Imbens (The Applied Econometrics Professor and Professor of Economics at Stanford Graduate School of Business, United States).
Professor Guido Imbens taught the 2018 Tinbergen Institute Econometrics lectures on May 30 – June 1. Most famous for his work on developing methods to draw causal inference, he is currently also very much interested in using machine learning techniques. Naturally, the lectures covered both topics.
Many thanks for teaching this year’s econometrics lectures! A great many students and staff members are very excited about your visit. How about you: happy to be back in Rotterdam, where you started studying econometrics?
I’m very happy to be back here. I have been back here before for seminars, but never actually to give some lectures. It has been very pleasant. The students have been very impressive. Some of the faculty I took courses with in the 1980s are still here, including for instance Casper de Vries.
To what extent were you inspired by Jan Tinbergen and Tjalling Koopmans (two Dutch econometricians and Nobel laureates) to start studying econometrics?
Tinbergen has been a great source of inspiration. When I was in high school thinking what to study, someone showed me a small Dutch book by Tinbergen on econometrics, which motivated me to study econometrics. Later when I was a student here in Rotterdam I attended some public lectures by him. Much later I read his early work on instrumental variables, translated in the Hendry and Morgan volume, with much appreciation. I wrote a book review stressing how close the Tinbergen and Haavelmo perspective is to the modern potential outcome approach to causality. Koopmans’ work is also very impressive, but his approach, and in general the Cowles commission approach to econometrics that he was involved in does not resonate quite as much with me. I also like the way Tinbergen was very involved in empirical work and policy questions.
Hendry, D.F., and M.S. Morgan, eds. The foundations of econometric analysis. Cambridge University Press, 1997.
Guido Imbens, Book Review of “The Foundations of Econometric Analysis”, by David Hendry and Mary Morgan, Journal of Applied Econometrics
Your lecture is about machine learning and causal inference. Machine learning techniques are often used for the purpose of prediction, whereas economists are arguably more interested in establishing causality. What can we, as economists, learn from machine learning techniques? And, taking this a step further, do you think that in the future, machine learning techniques can replace (quasi-)experimental methods to establish causal patterns?
I agree that economists are more interested in causality, although some problems in economics can be cast directly as prediction problems. Mullainathan and Spiess (2018) argue that prediction tasks and causal questions are often intimately linked. When we are interested in causal questions we typically need to modify machine learning methods to ensure that they are answering the questions we are interested in. Susan Athey (2017) wrote a nice paper on this. That does not change the fact that we have a lot to learn from this literature. A huge amount of progress has been made on these prediction problems, and that is going to affect much of what we do in econometrics. Regarding your final question, I don’t think these methods will simply replace current quasi-experimental methods. Instead, they will enhance them.
Mullainathan, S., and J. Spiess (2017). “Machine learning: an applied econometric approach.” Journal of Economic Perspectives
Athey, S. (2017). “Beyond prediction: Using big data for policy problems.” Science
And how about the other way around: would you say data scientists can learn a lot from the techniques economists use to identify causal effects? And if so, how?
Definitely. I think data scientists are increasingly realizing that causal questions are quite different from prediction questions, and there is much interaction between data scientists and economists/econometricians to exploit insights about causality from those disciplines. I see that all the time at Amazon where I have spent part of my time in recent years. It is actually very exciting to see these collaborations between computer scientists, statisticians, and economists at the tech companies. There truly is much to be gained from those interactions.
Some economists, most famously James Heckman and Angus Deaton, have argued that the focus on identification in economics research has gone “too far”. In a famous reply, you defended the use of (quasi-)experimental methods, but did express some concern. In particular, you argued that the trend towards credible causal inference might lead researchers away from questions where randomization is difficult or impossible. Now, almost a decade later, to what extent do you think this fear has materialized?
No, I was never worried about this, and this fear has not materialized at all. I think the trend towards more credible causal inference has continued to improve empirical practice. Empirical work is so much better now than it was in the seventies and eighties, as a result of what I see as the movement started by the Princeton labor economists, including Orley Ashenfelter, David Card, Alan Krueger, Bob Lalonde, and Josh Angrist. Recently, Deaton revisited some of these issues, in a joint paper with Nancy Cartwright. A bunch of people, including myself, wrote comments on their paper. It is somewhat frustrating. In the end I do not really understand what the point is that Deaton is trying to make. His claim in both papers that there is in the end nothing special about randomized experiments makes little sense to me, and, I think, to most people. For instance, the FDA (Food and Drug Administration) insists on using insights obtained from experiments. Also at the tech companies, including Google, Facebook, Amazon, and others, randomized experiments are widely used, precisely because they are viewed as so much more credible than other research designs. This has led to exciting new experimental designs, such as the multi-armed bandits that I discussed in the course, and it is motivating much new research, for example finding ways of effectively combine experimental and observational data. See for example the paper with Susan Athey, Raj Chetty and Hyunseung Kang.
Athey, S., Chetty, R., Imbens, G., & Kang, H. (2016). Estimating Treatment Effects using Multiple Surrogates: The Role of the Surrogate Score and the Surrogate Index.
Deaton, A. Instruments of development: Randomization in the tropics, and the search for the elusive keys to economic development. No. w14690. National Bureau of Economic Research, 2009.
Deaton, A., and N. Cartwright (2018). “Understanding and misunderstanding randomized controlled trials.” Social Science & Medicine.
Heckman, James J., and Sergio Urzua. “Comparing IV with structural models: What simple IV can and cannot identify.” Journal of Econometrics 156.1 (2010): 27-37.
Imbens, G. (2018). Comments on “Understanding and misunderstanding randomized controlled trials.”
Imbens, Guido W. “Better LATE than nothing: Some comments on Deaton (2009) and Heckman and Urzua (2009).” Journal of Economic literature 48.2 (2010): 399-423.
You are a very successful and influential researcher and – according to many – a potential successor of Jan Tinbergen and Tjalling Koopmans (the two Dutch Nobel laureates in economics). What advice would you give to PhD students and other young researchers?
Do I have any advice? That is a great question. Actually I do! When I was fresh out of graduate school, and a first year assistant professor at Harvard, my colleague Josh Angrist would drag me to the labor seminars every week, telling me I should listen to the empirical researchers. That advice is even more relevant now. Economics has become a very empirical discipline, and you should read and study the leading empirical researchers, such as my colleague Raj Chetty, David Card, Esther Duflo, Amy Finkelstein, Emmanuel Saez, and others. Study what questions they focus on, what methods they use, what empirical strategies they employ, and what makes their work both interesting and convincing. This is true both if you want to do empirical work yourself, or if you want to do econometric theory. In the former case you want to think through what makes that work of interest to a larger group of researchers. Maybe you can study similar questions in a different context or country. Maybe you can apply the same methodologies to different questions. If you do econometric methodology you want to make sure that the methods are going to be of interest to empirical researchers, and the best way to ensure that is to see what those researchers are doing, and where methods may be improved.