NWO Veni grant awarded to Phyllis Wan
The Netherlands Organisation for Scientific Research (NWO) has awarded research fellow Phyllis Wan of Erasmus School of Economics with an individual NWO Veni grant.
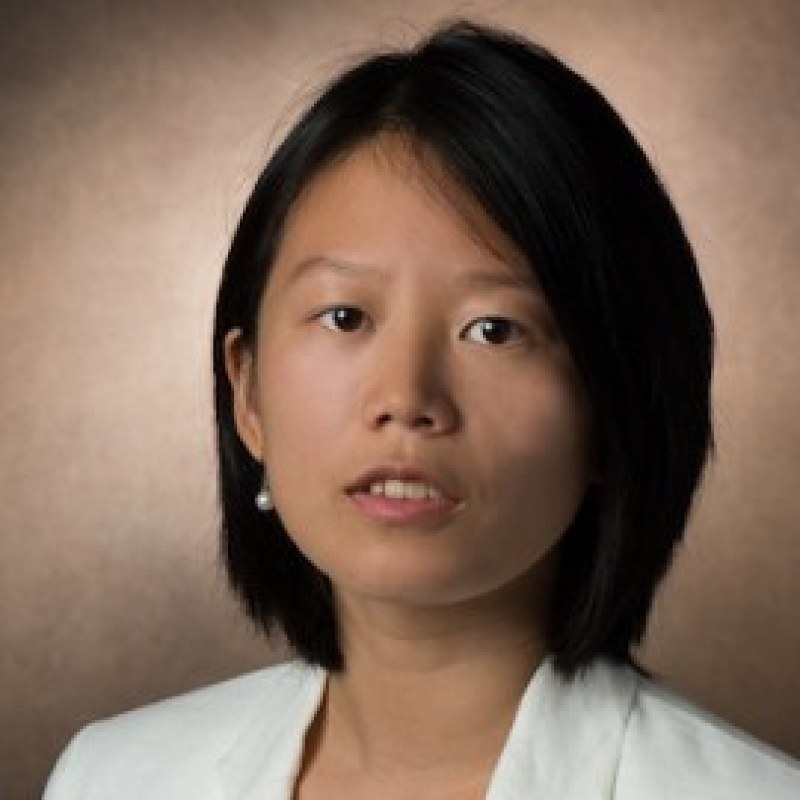
The NWO Veni grant, of up to 280.000 euros, is awarded to excellent researchers who have recently obtained their PhD to conduct independent research and develop their ideas for a period of three years. Laureates are at the start of their scientific career and display a striking talent for scientific research.
Phyllis holds a PhD from Columbia University, United States and her research interest lies in the study of complex data structures, such as networks and time series. Her recent research focuses on extreme value analysis and its applications in economics, finance, meteorology and hydrology.
Phyllis’s three-year NWO Veni project is called “Data-driven pattern recognition in multivariate extremes” and focusses on the study of extremes. Extreme events, such as the 2008 financial crisis or the 2021 European flooding, entail high risks for the society. Preventing and responding to these catastrophic outcomes rely on quantifying the risks of extreme scenarios. However, as extreme observations are scarce by nature, modelling their behaviour is challenging especially as the dimension of the data increases. Effective modelling relies on the detection of patterns in the data. This research aims at building effective data-driven pattern recognition methodologies for extremes.
Read more on the website of Erasmus School of Economics.