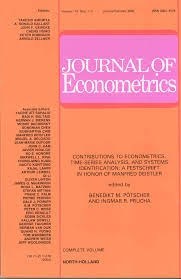
Blasques Albergaria Amaral, F., Koopman, S., Mallee, M. and Zhang, Z. (2016). Weighted Maximum Likelihood for Dynamic Factor Analysis and Forecasting with Mixed Frequency Data Journal of Econometrics, 193(2):405--417.
-
Affiliated authors
-
Publication year2016
-
JournalJournal of Econometrics
For the purpose of forecasting key macroeconomic or financial variables from a panel of time series variables, we adopt the dynamic factor model and propose a weighted likelihood-based method for parameter estimation. The loglikelihood function is split into two parts that are weighted differently. The first part is associated with the key variables while the second part is associated with the related variables which may contribute to the forecasting of key variables. We derive asymptotic properties, including consistency and asymptotic normality, of the weighted maximum likelihood estimator. We show that this estimator outperforms the standard likelihood-based estimator in approximating the true unknown distribution of the data as well as in out-of-sample forecasting accuracy. We verify the new estimation method in a Monte Carlo study and investigate the role of different weights in different settings. In the context of forecasting gross domestic product growth, this key variable is typically observed at a low (quarterly) frequency while the supporting variables are observed at a high (monthly) frequency. We adopt a low frequency representation of the mixed frequency dynamic factor model and discuss the computational efficiencies of this approach. In our empirical study for the U.S. economy, we present improvements in nowcasting and forecasting accuracy when the weighted likelihood-based estimation procedure is adopted.