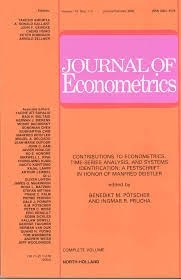
Kleibergen, F. (2004). Invariant Bayesian Inference in Regression Models that is robust against the Jeffreys-Lindleys Paradox Journal of Econometrics, 123(2):227--258.
-
Affiliated author
-
Publication year2004
-
JournalJournal of Econometrics
We obtain the prior and posterior probability of a nested regression model as the Hausdorff-integral of the prior and posterior on the parameters of an encompassing linear regression model over a lower-dimensional set that represents the nested model. The Hausdorff-integral is invariant and therefore avoids the Borel-Kolmogorov paradox. Basing priors and prior probabilities of nested regression models on the prior on the parameters of an encompassing linear regression model reduces the discrepancies between classical and Bayesian inference, like, the Jeffreys-Lindley's paradox. We illustrate the analysis with examples of linear restrictions, i.e. a linear regression model, and non-linear restrictions, i.e. a cointegration and an autoregressive moving average model, on the parameters of an encompassing linear regression model.