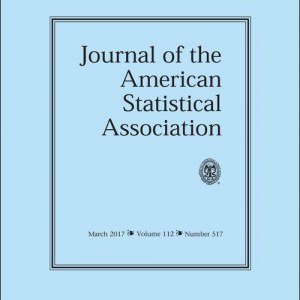
He, Y. (2024). Ridge Regression Under Dense Factor Augmented Models Journal of the American Statistical Association, 119(546):1566--1578.
-
Affiliated author
-
Publication year2024
-
JournalJournal of the American Statistical Association
This article establishes a comprehensive theory of the optimality, robustness, and cross-validation selection consistency for the ridge regression under factor-augmented models with possibly dense idiosyncratic information. Using spectral analysis for random matrices, we show that the ridge regression is asymptotically efficient in capturing both factor and idiosyncratic information by minimizing the limiting predictive loss among the entire class of spectral regularized estimators under large-dimensional factor models and mixed-effects hypothesis. We derive an asymptotically optimal ridge penalty in closed form and prove that a bias-corrected k-fold cross-validation procedure can adaptively select the best ridge penalty in large samples. We extend the theory to the autoregressive models with many exogenous variables and establish a consistent cross-validation procedure using the what-we-called double ridge regression method. Our results allow for nonparametric distributions for, possibly heavy-tailed, martingale difference errors and idiosyncratic random coefficients and adapt to the cross-sectional and temporal dependence structures of the large-dimensional predictors. We demonstrate the performance of our ridge estimators in simulated examples as well as an economic dataset. All the proofs are available in the supplementary materials, which also includes more technical discussions and remarks, extra simulation results, and useful lemmas that may be of independent interest.