Paper by Rosnel Sessinou has been published in the Journal of Business & Economics Statistics
The paper 'Precision Least Squares: Estimation and Inference in High-Dimensions' by Candidate Fellow Rosnel Sessinou (Erasmus University Rotterdam), in collaboration with Luca Margaritella (Lund University, Sweden), has been published online in the Journal of Business & Economics Statistics.
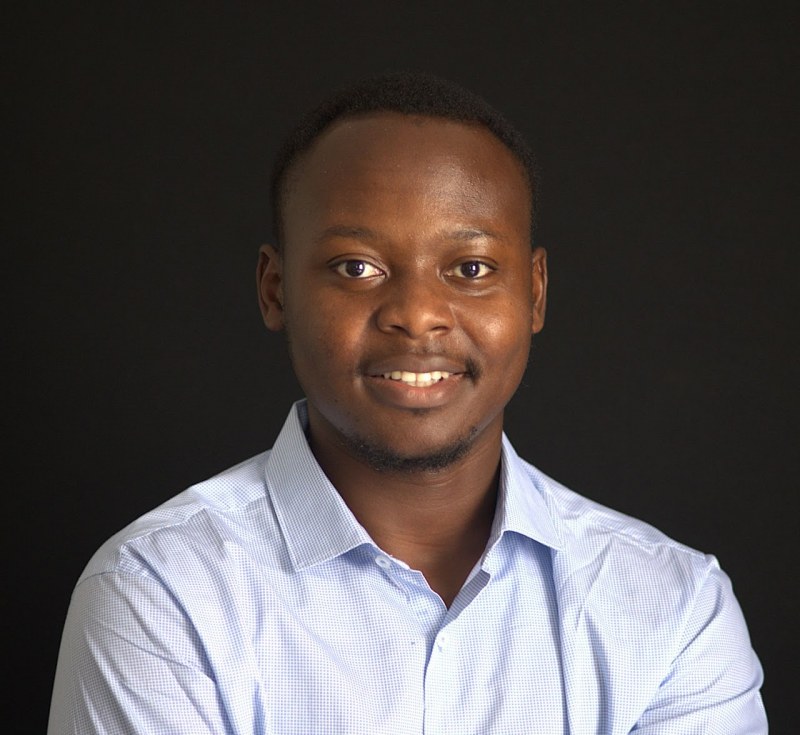
Abstract
The least squares estimator can be cast as depending only on the precision matrix of the data, similar to the weights of a global minimum variance portfolio. We give conditions under which any plug-in precision matrix estimator produces an unbiased and consistent least squares estimator for stationary time series regressions, in both low- and high-dimensional settings. Such conditions define a class of “Precision Least Squares” (PrLS) estimators, which are shown to be approximately Gaussian, efficient, and to provide automatic family-wise error control in large samples. For estimating high-dimensional sparse regression models, we propose a LASSO Cholesky estimator of the plug-in precision matrix. We show its consistency and how to properly bias correct it, thereby obtaining a LASSO Cholesky-based PrLS (LC-PrLS) estimator. LC-PrLS performs well in finite samples and better than state-of-the-art high-dimensional estimators. We employ LC-PrLS to investigate the dynamic network of predictive connections among a large set of global bank stock returns. We find that crisis years correspond to a collapse of predictive linkages.
Article citation
Luca Margaritellas and Sessinou, Rosnel, 'Precision Least Squares: Estimation and Inference in High-Dimensions'Journal of Business & Economics Statistics, doi.org/10.1080/07350015.2024.2440573.